Forecast by relying on statistics and probabilities
Every forecast relies on statistical methods and calculated probabilities. This means that:
- a good forecast requires a large quantity of data
- a forecast is never a certainty.
In the first instance, seeking to forecast the malfunction date of a machine or part will therefore involve accepting to allow the machines to fail in order to obtain as many instances of malfunction as possible so as to integrate them into the statistical analysis model, and then using this model to calculate a theoretical malfunction date… We keep in mind that whatever the reliability of the model, this date will always include a margin of error and in the case of industrial equipment, the margin of error may be greater because each machine is more or less a separate instance.
This approach is too costly and risky to be acceptable.
Anticipate to allow more time
We prefer anticipation over forecasting, by using a mix of conditional and predictive methods, and by placing emphasis on the significance and reliability of diagnosis.
We developed our automated diagnostic algorithms so that maintenance teams can be alerted only when a machine actually has a fault, but sufficiently early so that repairs can be planned in a calm manner.
Our objective is not so much to forecast the outcome (occurrence of malfunction) but to identify the trigger element at the earliest possible time. In this way, we prolong the interval between the time of diagnosis and the time at which the critical level is reached and we thereby provide a longer timeframe during which the intervention can be carried out.
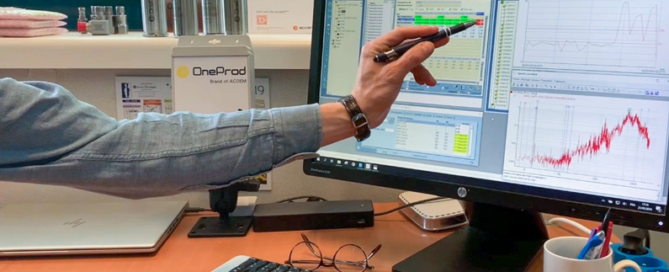
To predict means to announce an event by intuition or premonition, without real proof, whereas to forecast is to believe that a future event is very probable based on some data. It is understandable that the NF EN 13306 Standard does not recognise the term “predictive maintenance”.
Therefore, with the development of artificial intelligence (particularly non-supervised machine learning), it is possible – from a collection of data – to “read” disparate indices which would not attract attention when considered in isolation but “reveal” a problem when brought together.
Future generations of algorithms will make it possible to give automated diagnostic tools this element of intuition which is sometimes used by experienced specialists to solve complex problems. Their predictions will make it possible for us to broaden the scope of our forecasts.
(To know more about this, read our article: How does artificial intelligence improve vibration analysis?).
We see predictive maintenance as a science that involves the detection of weak signals and extrapolation of trend curves for diagnostic purposes, and not as a probabilistic method for calculating maintenance dates. What about you?